This chapter should be cited as follows:
Buekens P, Berrueta M, Glob Libr Women's Med
ISSN: 1756-2228; DOI 10.3843/GLOWM.417533
The Continuous Textbook of Women’s Medicine Series – Obstetrics Module
Volume 17
Maternal immunization
Volume Editors:
Professor Asma Khalil, The Royal College of Obstetricians and Gynaecologists, London, UK; Fetal Medicine Unit, Department of Obstetrics and Gynaecology, St George’s University Hospitals NHS Foundation Trust, London, UK
Professor Flor M Munoz, Baylor College of Medicine, TX, USA
Professor Ajoke Sobanjo-ter Meulen, University of Washington, Seattle, WA, USA
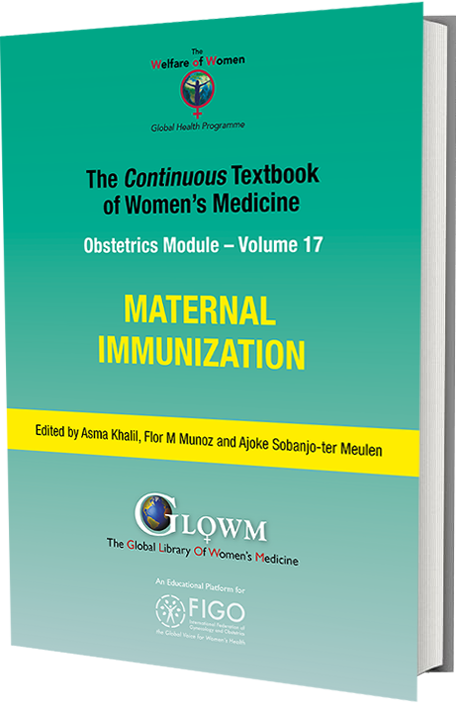
Chapter
Maternal and Neonatal Disease Surveillance Systems for Vaccine Preventable Diseases
First published: May 2023
Study Assessment Option
By completing 4 multiple-choice questions (randomly selected) after studying this chapter readers can qualify for Continuing Professional Development awards from FIGO plus a Study Completion Certificate from GLOWM
See end of chapter for details

INTRODUCTION
There is a very long tradition of registering pregnant persons and births. Collecting data during pregnancy and childbirth is feasible as most pregnant persons attend prenatal care and deliver with skilled birth attendants. UNICEF estimated that between 2015 and 2020 87% of pregnant persons in the world had at least one prenatal visit, and 83% of births were attended by skilled attendants.1,2 This is the case in most low- and middle-income countries (LMICs), such as the Democratic Republic of the Congo (DRC) where, in 2018, 82% had at least one prenatal visit, 85% of births were attended by a skilled birth attendant and 82% of deliveries took place in a health facility.1,2
Prenatal care and delivery room registries have been used in paper form for many years. Evidence suggests that maternity registries are a reliable data source because of their consistency with medical records. In 2000, we found that in three departments in Guatemala the date of delivery coincided in 96.2% of cases where it was recorded in both delivery room registries and medical records; the person who attended the delivery coincided in 97.8% of cases; and cesarean sections, maternal complications, and neonatal deaths coincided in 100% of cases.3 Data from sentinel prenatal clinics are often used to generate population-based estimates of key indicators, such as HIV/AIDS seroprevalence.4 Perinatal data collection systems have evolved from registries to more detailed and structured perinatal records. Perinatal databases were among the first ones to be digitized, with electronic perinatal databases appearing in the early 1980s.5
Our objectives are to review perinatal information systems and health indicators. We define the perinatal period as including pregnancy, and/or delivery, and/or the neonatal period (0–28 days). Some systems may include follow-up data beyond the neonatal period. We will also summarize data analysis methods, with an emphasis on the choice of comparison groups and potential biases.
PERINATAL INFORMATION SYSTEMS
We performed a landscape analysis to identify perinatal information systems, which could inform maternal immunization active safety surveillance in LMICs. The landscape analysis included a scoping review, expert consultation, and interviews of key informants.6 The scoping review found eight eligible information systems that included research networks (Global Network’s Maternal Newborn Health Registry (GN-MNHR)7,8 and International Network for the Demographic Evaluation of Populations and their Health (INDEPTH)),9,10,11 perinatal electronic medical records (Perinatal Informatic System (SIP),12,13,14,15 Pregnancy Exposure Registry & Birth Defects Surveillance (PER/BDS)),16 and SmartCare,17,18 or custom software platforms for health information systems (District Health Information Software 2 (DHIS 2),19,20,21,22 Open Medical Record System (OpenMRS),23,24,25,26 and Open Smart Register Platform (OpenSRP))27,28,29 (Table 1). These are active and existing systems being used in LMICs in Africa, Asia, and Latin America that can collect individual-level perinatal data, at the facility and community levels, and have the capacity to inform background rates on adverse perinatal outcomes.
1
Scoping review – perinatal data collection systems. Data from Berrueta M, et al.6
Data collection system | Description | Countries serving to capture MNCH individual data | Number of pregnant women/deliveries registered | |
Research network | Global Network’s Maternal Newborn Health Registry | The Global Network’s Maternal Newborn Health Registry (MNHR) is a prospective, population-based registry of pregnancies at the Global Network sites. It started in 2008, enrolls and follows up on pregnant women and their newborns up to 42 days postpartum | Captures data in six countries, including sites in Pakistan; Kenya; Zambia; Democratic Republic of the Congo; Guatemala; and two in India | 370,000 mothers and their infants have been enrolled so far |
International Network for the Demographic Evaluation of Populations and their Health | INDEPTH is a network of independent Health and Demographic Surveillance System (HDSS) sites that carry out longitudinal research. The INDEPTH Network Maternal, Newborn & Child Health Working Group (MNCH-WG) coordinates the surveillance of pregnancies and outcome tracking | Bangladesh; Burkina Faso; Cote d’Ivoire; Ethiopia; Gambia; Ghana; Guinea-Bissau; India; Indonesia; Kenya; Malawi; Malaysia; Mozambique; Nigeria; Senegal; South Africa; Tanzania; Uganda; Vietnam | 97,499 estimated total births over 5 years (2012–2017) captured within the HDSS (only in five sites: Bandim; Matlab; Kintampo; Dabat; Igangamayuge) | |
Electronical medical record | Perinatal Informatic System | Perinatal Informatic System (“SIP”, by the acronym in Spanish: Sistema Informático Perinatal) by Pan American Health Organization (PAHO) is a perinatal clinical record that Ministries of health and maternity services (public and private) have adopted | Cuba; El Salvador; Guatemala; Honduras; Nicaragua; Panama; Dominician Republic; Mexico; Argentina; Bolivia; Brazil; Chile; Colombia; Ecuador; Paraguay; Peru; Uruguay; Venezuela | Not available |
Pregnancy Exposure Registry & Birth Defects Surveillance | PER/BDS is an electronic medical record system designed to collect data on pregnancy exposures during routine care | South Africa | 42,300 estimated deliveries per year in Gauteng and in Western Cape | |
SmartCare | SmartCare is a portable, integrated, and electronic medical system developed by Zambia's Ministry of Health | Zambia | Not available | |
Custom Software platform | District Health Information Software 2 tracker | DHIS2 is a customize platform typically used as a national health information system. The tracker module allows for the collection of data on pregnancies and babies and to track longitudinally the progress of patients over time | Bangladesh; Liberia; West Bank and Gaza; South Africa | Not available |
Open Medical Record System | OpenMRS is a software platform and a reference application, which enables the design of a customized medical records system with no programming knowledge | Uganda; Malawi; Rwanda; Lesotho; Kenya; Haiti | Not available | |
Open Smart Register Platform | Open Smart Register Platform is a mobile-first platform, built to enable data-driven decision-making at all levels of the health system with the following health modules: reproductive, maternal, neonatal, and child health, childhood immunizations, tuberculosis, nutrition, malaria, and early childhood development | Indonesia; Pakistan | Not available |
These systems are supported by different types of institutions30 such as private foundations (e.g., Bill & Melinda Gates Foundation), governmental agencies in high-income countries (e.g., United States Agency for International Development (USAID), Centers for Disease Control and Prevention, NORAD), global health initiatives (e.g., The Global Alliance for Vaccines and Immunization), research institutes (e.g., National Institute of Child Health and Human Development), non-governmental organizations (e.g., Comic Relief), international organizations (e.g., World Health Organization (WHO)/Pan American Health Organization), universities (e.g., University of Oslo), private sector organizations (e.g., GlaxoSmithKline), and LMIC governments (e.g., South Africa National Department of Health). Some organizations were also responsible for the development and implementation of the systems and for its optimal operability, such as the University of Oslo in the case of DHIS 2.
Each information system uses its own procedures to collect data, follow documented manuals, and certify the training of users and administrators. DHIS 2, GN-MNHR, and INDEPTH were selected as the top three systems after experts reviewed the governance, system management, system design, data source, data management, and data quality domains of each system in an expert consultation. The selected systems demonstrate features that support the protection and privacy of collected information through anonymization of data, implementation of passwords before access, or external security (cybersecurity), and internal and external quality control procedures. The three systems can collect patient data and longitudinally track pregnant persons’ progress and their babies over the prenatal and postnatal periods. However, the timing of capturing information from antenatal visits is different among the systems. GN-MNHR and INDEPTH collect antenatal care data retrospectively, GN-MNHR collects their data at enrollment and delivery, and INDEPTH collects self-reported data retrospectively from household visits. It is important to note that INDEPTH has been adapted to collect data prospectively in Burkina Faso, Kenya, and Mozambique to evaluate artemisinin exposure and monitoring in pregnant people.31
PERINATAL HEALTH INDICATORS
The Global Alignment of Immunization Safety Assessment in pregnancy (GAIA) project32 and the WHO Global vaccine safety in pregnancy multi-country collaborative study (MCC) have started to define and pilot case definitions for priority perinatal outcomes. The GAIA partners developed the first set of over 29 standardized case definitions of prioritized obstetric and neonatal outcomes based on the standard Brighton Collaboration process.
The feasibility to implement GAIA variables in the field remains an open question.32,33 One challenge is to define what is the minimum acceptable set of variables that are needed to have quality information about perinatal outcomes and to inform maternal immunization active surveillance systems during pregnancy. As part of our landscape analysis, experts were invited to select the minimum set of variables, or maternal and neonatal outcomes, that should be collected in routine perinatal systems in LMICs to monitor COVID-19 vaccine post-marketing surveillance during pregnancy (Table 2).34
2
Prioritized maternal, neonatal, and vaccine-related variables for COVID-19 vaccines active safety surveillance. Data from Pingray V, et al.34
Variable (votes) | ||
Maternal | Neonatal outcomes | Vaccine-related variables |
Maternal death (14) | Neonatal death (14) | Date of vaccination (14) |
Spontaneous abortion (12) | Preterm birth (14) | Vaccine lot number (13) |
Intrauterine growth retardation (11) | Stillbirth (13) | Name of vaccination (13) |
Hypertensive disorders of pregnancy (9) | Congenital anomalies (13) | Gestational age at vaccination (11) |
Threatened preterm labor (8) | Low birth weight (13) | Dosage (9) |
Maternal pneumonia (8) | Small for gestational age (12) | Vaccine expiration date (7) |
Postpartum hemorrhage (7) | Respiratory distress (11) | |
Maternal age (7) | Live birth (9) | |
Chorioamnionitis (7) | Neurodevelopmental delay (7) | |
Microcephaly (7) | ||
Enabling term | ||
Assessment of gestational age (10) |
Note: variables prioritized by 80% of the experts' panel are highlighted in bold.
This panel’s prioritization of maternal, neonatal, and vaccine-related variables can guide the necessary adaptation of existing maternal and neonatal data-collection systems in LMICs for maternal vaccine active safety surveillance. Among the eight perinatal information systems identified by our landscape analysis, we can see that all systems collect vital data, such as maternal and neonatal deaths (Tables 3 and 4). The most frequently recorded perinatal outcomes are fetal distress, postpartum hemorrhage, antenatal bleeding, dysfunctional labor, spontaneous abortion, congenital anomalies, neonatal infections, preterm birth, stillbirth, low birth weight, small for gestational age, and respiratory distress. Some outcomes are not currently recorded by any of the selected systems (i.e., premature preterm rupture of membranes, preterm labor, insufficient cervix, and neurodevelopmental delay).
3
Maternal outcomes registered in perinatal systems. Data from Berrueta M, et al.6
Variable | PER/ | |||||||
Maternal death | Yes | Yes | Yes | Yes | Yes | Yes | Yes | Yes |
Pre-eclampsia/ | Yes | Yes | No | Yes | N/A | Yes | N/A | N/A |
Gestational hypertension | N/A | Yes | Yes | N/A | N/A | N/A | N/A | N/A |
Fetal distress | No | No | Yes | Yes | Yes | Yes | Yes | N/A |
Ectopic pregnancy | No | Yes | No | N/A | N/A | N/A | N/A | N/A |
Postpartum hemorrhage | Yes | Yes | Yes | Yes | Yes | Yes | Yes | N/A |
Spontaneous abortion | Yes | Yes | Yes | Yes | Yes | Yes | Yes | N/A |
Antenatal bleeding | No | Yes | Yes | Yes | Yes | Yes | Yes | N/A |
Dysfunctional labor | Yes | Yes | No | Yes | Yes | Yes | Yes | N/A |
Fetal growth retardation | Yes | No | No | Yes | N/A | Yes | Yes | N/A |
Gestational diabetes | Yes | No | No | No | Yes | Yes | N/A | N/A |
Postpartum endometritis | N/A | Yes | No | No | Yes | Yes | N/A | N/A |
Chorioamnionitis | N/A | No | No | No | Yes | Yes | N/A | N/A |
Premature preterm rupture of membranes | No | No | No | N/A | N/A | N/A | N/A | N/A |
Preterm labor | No | No | No | N/A | N/A | N/A | N/A | N/A |
Insufficient cervix | No | No | No | N/A | N/A | N/A | N/A | N/A |
N/A, Information not available.
Variability in case definitions and diagnostic criteria across data sources and among different cultures and languages is a challenge. The lack of harmonization of case confirmation/classification among systems is also a defined problem. These eight systems are not currently using the harmonized case definitions developed by the Brighton Collaboration GAIA project,35 however, some of them (e.g., GN-MNHR) follow the WHO perinatal definitions and others (i.e., DHIS 2, INDEPTH, OpenMRS, SIP, and PER/BDS) are utilizing the tenth revision of the International Classification of Diseases (ICD10) for coding outcomes and conditions.
GN-MNHR and INDEPTH coordinate the data collection and validation across their sites. In contrast, DHIS 2 offers different modules on an open-source platform that each site can customize, modify, and adapt for use with total autonomy. However, all of them have the flexibility to add new variables.
DATA ANALYSIS
Comparison groups
The choice of a comparison group is a key step in the analysis of vaccine outcomes. The analyses compare vaccinated ('exposed') to non-vaccinated ('non-exposed') pregnant persons. Epidemiologists classify subjects enrolled in cohort studies as 'exposed' and 'non-exposed' to avoid confusion with 'cases' and 'controls' enrolled in retrospective case-control studies that compare persons with and without a health condition.36
It is important to have high-quality data for both the vaccinated and non-vaccinated persons. Several variables, often listed as potential adverse events, are not rare and are expected to occur frequently among non-exposed. For example, the INTERGROWTH-21st study found 5.5% of all newborns in urban populations in eight countries were low birthweight (LBW) newborns.37 Thus, it is not sufficient to collect high-quality data among the non-vaccinated. Comparisons to vaccinated persons can be affected by measurement biases if the quality of the data is not homogenous. For this reason, it may be easier to interpret perinatal databases covering all pregnant individuals than active safety surveillance studies only collecting data among vaccinated persons.
Vaccine studies often compare frequencies of health outcomes among the exposed to background rates in the general population. In practice, background rates generally differ between different populations and different data-collection systems. For frequent events, such as LBWs, data are often only available in LMICs from surveys, such as the Demographic and Health Surveys.38 Data might not be available for less frequent adverse events in LMICs. Thus, comparisons to background rates are often imprecise and can only detect very large differences. Analyses comparing vaccinated and non-vaccinated pregnant persons from the same perinatal database are much more likely to provide precise estimates than comparisons to background rates from the general population. As seen above, the long-standing tradition of systematically collecting data during pregnancy provides a unique opportunity to compare vaccinated and non-vaccinated persons.
Biases
The fundamental characteristic of perinatal data, the length of gestation, needs to be considered. The likelihood to be vaccinated increases with the length of gestation (Figure 1). Crude data will thus give the impression that vaccines 'protect' against preterm delivery and other adverse birth outcomes. Influenza vaccine studies have shown that not adjusting for length of gestation can bias estimates in favor of vaccination by 10–26%.39 The analysis should also consider the gestational age at which the vaccination is performed. Epidemiologists see the time before vaccination as 'immortal time' because death occurring during that time would have made vaccination impossible.39 Death and other adverse events (e.g., preterm delivery) occurring during the 'immortal time' would thus erroneously be attributed to the absence of the vaccine. Availability of vaccines can also vary with time, which could create additional biases with persons delivering early having a smaller likelihood of still being pregnant when vaccinations become available.39 The key point is to take time into account.
1
Pregnant person A delivers at 40 weeks and is vaccinated. Pregnant person B delivers at 30 weeks and has a lower likelihood to be vaccinated.
Potential confounding variables also need to be taken into account. There might be a healthy vaccine bias, as a higher socio-economic status is associated with both vaccination status and better health outcomes. On the other hand, persons with comorbidities might be vaccinated more often, which would create a non-causal association between vaccination and poor health outcomes. One approach to adjust for both time and potential confounders is to perform multivariable analyses. A Cox regression model is often used because it gives an adjusted hazard ratio, which can be interpreted as a relative risk. One example, shown in Table 5, from Zerbo and collaborators, demonstrates the misleading effect of crude data, which gives the impression that the influenza vaccine protects against preterm birth and LBW.40 Crude risk ratios were statistically significantly lower than 1. This effect disappears after performing a Cox regression, with adjusted hazard ratios not statistically significantly different from 1.
5
Maternal vaccination during pregnancy and birth outcomes. Adapted from Zerbo et al.40
Outcomes | Vaccinated N = 64,748 | Non-vaccinated N = 81,119 | Crude risk ratio | Adjusted hazard ratio |
Preterm birth | 4,183 (6.5) | 6,740 (8.3) | 0.78 (0.75–0.81) | 0.98 (0.93–1.03) |
Low birthweight | 3,242 (5.0) | 5,128 (6.3) | 0.79 (0.76–0.83) | 0.95 (0.86–1.06) |
*Cox regression models with vaccination status as a time-dependent variable adjusted for potential confounders.
CONCLUSION
A significant number of perinatal information systems are active in LMICs. They provide a unique opportunity to collect data in both vaccinated and non-vaccinated populations. Data are limited to minimum basic data sets, but systems often allow additional variables to be added. The data analysis needs to take length of gestation and other potential confounders into account.
PRACTICE RECOMMENDATIONS
- Perinatal information systems are often available to monitor perinatal outcomes in LMICs.
- Routine data collected in perinatal information systems can provide background perinatal outcome rates for vaccine active safety surveillance.
- Variability in case definitions and diagnostic criteria across data sources and countries is clearly a challenge.
- Adjusting for length of gestation and other potential confounders is essential.
ACKNOWLEDGEMENTS
This work was supported, in part, by the Bill & Melinda Gates Foundation [INV008443].
We thank Erin Goucher for her editorial assistance.
CONFLICTS OF INTEREST
The author(s) of this chapter declare that they have no interests that conflict with the contents of the chapter.
Feedback
Publishers’ note: We are constantly trying to update and enhance chapters in this Series. So if you have any constructive comments about this chapter please provide them to us by selecting the "Your Feedback" link in the left-hand column.
REFERENCES
UNICEF. Antenatal Care 2021 [Available from: https://data.unicef.org/topic/maternal-health/antenatal-care/]. | |
UNICEF. Global Databases: Institutional deliveries 2021 [Available from: https://data.unicef.org/topic/maternal-health/delivery-care/]. | |
Barillas E, Valladares R, Jaime R, et al. Assessment of the Quality of Maternity Registers in Guatemala. Chapel Hill, NC: MEASURE Evaluation, 2001. | |
Eaton JW, Rehle TM, Jooste S, et al. Recent HIV prevalence trends among pregnant women and all women in sub-Saharan Africa: implications for HIV estimates. AIDS 2014;28(Suppl 4):S507–14. | |
Sokol RJ, Chik L. Perinatal computing. An overview. Acta Obstet Gynecol Scand Suppl 1982;109:7–10. | |
Berrueta M, Ciapponi A, Bardach A, et al. Maternal and neonatal data collection systems in low- and middle-income countries for maternal vaccines active safety surveillance systems: A scoping review. BMC Pregnancy and Childbirth 2021;21(1):217. | |
RTI: Research Triangle Institute Research Triangle Park, NC: RTI International, 2021 [Available from: https://www.rti.org/] [Accessed 15 Oct 2021]. | |
The Global Network for Women’s and Children’s Health Research. Foundation for the National Institutes of Health, 2020. Available from: https://globalnetwork.azurewebsites.net/ [Accessed 14 Oct 2021]. | |
Baschieri A, Gordeev VS, Akuze J, et al. "Every Newborn-INDEPTH" (EN-INDEPTH) study protocol for a randomised comparison of household survey modules for measuring stillbirths and neonatal deaths in five Health and Demographic Surveillance sites. Journal of global health 2019;9(1):010901-. | |
Tesfaye G, Loxton D, Chojenta C, et al. Magnitude, trends and causes of maternal mortality among reproductive aged women in Kersa health and demographic surveillance system, eastern Ethiopia. BMC Women's Health 2018;18(1):198. | |
INDEPTH Network Accra: INDEPTH, 2021 [Available from: http://www.indepth-network.org/] [Accessed 14 Oct 2021]. | |
Sistema Informático Perinatal: Centro Latinoamericano de Perinatologia, Salud de la Mujer y Reproductiva (CLAP/SMR)-Montevideo; [Available from: http://www.clap.ops-oms.org/] [Accessed 14 Oct 2021]. | |
Fescina R. History of the Perinatal Information System. Making Pregnancy Safer 2010(8):1–8. | |
Karolinski A, Mercer R, Bolzán A, et al. Bases para el desarrollo e implementación de un modelo de información en salud de la mujer y perinatal orientado a la gestión en Latinoamérica. Revista Panamericana de Salud Publica 2018;42:1–11. | |
Serruya SJ, Duran P, Martinez G, et al. Maternal and congenital syphilis in selected Latin America and Caribbean countries: A multi-country analysis using data from the Perinatal Information System. Sexual Health 2015;12(2):164–9. | |
Mehta UC, van Schalkwyk C, Naidoo P, et al. Birth outcomes following antiretroviral exposure during pregnancy: Initial results from a pregnancy exposure registry in South Africa. South Afr J HIV Med 2019;20(1):971. | |
Mweebo K, editor Security of electronic health records in a resource limited setting: The case of smart-care electronic health record in Zambia. 3rd Australian eHealth Informatics and Security Conference Held on the 1–3 December, 2014 at Edith Cowan University, Joondalup Campus, Perth, Western Australia, 2014 SRI Security Research Institute, Edith Cowan University. | |
Gumede-Moyo S, Todd J, Bond V, et al. A qualitative inquiry into implementing an electronic health record system (SmartCare) for prevention of mother-to-child transmission data in Zambia: a retrospective study. BMJ open 2019;9(9):e030428. | |
DHIS2 District Health Information Software 2: University of Oslo; Norad; Norway. Research Council; PEPFAR; The Global Fund; [Available from: https://www.dhis2.org/] [Accessed 16 Oct 2021]. | |
Rawlins B. Measurement Matters! Improving Routine RMNCAH Data for Better Outcomes. Washington, DC: Unites States. USAIDS. Maternal and Child Survival Program, 2019. Available from: https://www.mcsprogram.org/march-26th-measurement-matters-improving-routine-rmncah-data-for-better-outcomes/ [Accessed 14 Oct 2021]. | |
Hassan S, Vikanes A, Laine K, et al. Building a research registry for studying birth complications and outcomes in six Palestinian governmental hospitals. BMC Pregnancy Childbirth 2017;17(1):112. | |
Xiong K, Kamunyori J, Sebidi J. The MomConnect helpdesk: how an interactive mobile messaging programme is used by mothers in South Africa. (Special Issue: Digital health in South Africa: the case of MomConnect past, present, and future.). BMJ Global Health 2018;3(Suppl 2):e000578 17 ref. 2018. | |
OpenMRS : Open Medical Record System. https://openmrs.org/(2020). Accessed 19 june 2020 Carmel, IN: OpenMRS Inc, 2020. | |
Mamlin BW, Biondich PG, Wolfe BA, et al. Cooking up an open source EMR for developing countries: OpenMRS – a recipe for successful collaboration. AMIA Annu Symp Proc 2006:529–33. | |
Bashiri A, Ghazisaeedi M. Open MRS softwares: effective approaches in management of patients' health information. International Journal of Community Medicine and Public Health 2017;4(11):4. | |
Satti H, Motsamai S, Chetane P, et al. Comprehensive approach to improving maternal health and achieving MDG 5: report from the mountains of Lesotho. PLoS One 2012;7(8):e42700. | |
OpenSRP: Open Smart Register Platform. Geneva: World Health Organization, 2016 [software]. Available from: https://www.who.int/reproductivehealth/topics/mhealth/openspr/en/ [Accessed 12 Oct 2021]. | |
Kevin K, Inraini F, Ahmad Rafi J, et al. Midwife service coverage, quality of work, and client health improved after deployment of an OpenSRP-driven client management application in Indonesia. Proceedings of the 5th International Conference on Health Sciences (ICHS 2018), 2019. | |
Haddad SM, Souza RT, Cecatti JG. Mobile technology in health (mHealth) and antenatal care-Searching for apps and available solutions: A systematic review. Int J Med Inform 2019;127:1–8. | |
Footman K, Chersich M, Blaauw D, et al. A systematic mapping of funders of maternal health intervention research 2000–2012. Global Health 2014;10:72. | |
Tinto H, Sevene E, Dellicour S, et al. Assessment of the safety of antimalarial drug use during early pregnancy (ASAP): protocol for a multicenter prospective cohort study in Burkina Faso, Kenya and Mozambique. Reprod Health 2015;12:112. | |
Zuber PLF, Moran AC, Chou D, et al. Mapping the landscape of global programmes to evaluate health interventions in pregnancy: the need for harmonised approaches, standards and tools. BMJ Glob Health 2018;3(5):e001053. | |
Kochhar S, Bonhoeffer J, Jones CE, et al. Immunization in pregnancy clinical research in low- and middle-income countries – Study design, regulatory and safety considerations. Vaccine 2017;35(48 Pt A):6575–81. | |
Pingray V, Belizán M, Matthews S, et al. Using maternal and neonatal data collection systems for coronavirus disease 2019 (COVID-19) vaccines active safety surveillance in low- and middle-income countries: an international modified Delphi study. Gates Open Res 2021;5. | |
Kochhar S, Bonhoeffer J, Jones CE, et al. Immunization in pregnancy clinical research in low- and middle-income countries – Study design, regulatory and safety considerations. Vaccine 2017;35(48, Part A):6575–81. | |
Schulz KF, Grimes DA. Case-control studies: research in reverse. Lancet 2002;359(9304):431–4. | |
Villar J, Cheikh Ismail L, Victora CG, et al. International standards for newborn weight, length, and head circumference by gestational age and sex: the Newborn Cross-Sectional Study of the INTERGROWTH-21st Project. Lancet 2014;384(9946):857–68. | |
DHS M. Demographic and Health Surveys 2021 [Available from: http://www.measuredhs.com/. | |
Fell DB, Dimitris MC, Hutcheon JA, et al. Guidance for design and analysis of observational studies of fetal and newborn outcomes following COVID-19 vaccination during pregnancy. Vaccine 2021;39(14):1882–6. | |
Zerbo O, Modaressi S, Chan B, et al. No association between influenza vaccination during pregnancy and adverse birth outcomes. Vaccine 2017;35(24):3186–90. |
Online Study Assessment Option
All readers who are qualified doctors or allied medical professionals can now automatically receive 2 Continuing Professional Development credits from FIGO plus a Study Completion Certificate from GLOWM for successfully answering 4 multiple choice questions (randomly selected) based on the study of this chapter.
Medical students can receive the Study Completion Certificate only.
(To find out more about FIGO’s Continuing Professional Development awards programme CLICK HERE)
